Module 7: Leveraging AI in the CSM Role
Learning Objectives:
- Explore how AI can enhance customer engagement and analytics.
- Use generative AI for task automation, call transcription, and sentiment analysis.
- Balance automation with a human-centric approach to customer relationships.
Introduction
Artificial Intelligence (AI) is revolutionizing the Customer Success Manager (CSM) role. By automating repetitive tasks, analyzing vast datasets, and providing predictive insights, AI empowers CSMs to:
-
Scale their impact by managing larger customer portfolios.
-
Reduce churn through proactive interventions and personalized engagement.
-
Enhance decision-making with data-driven insights.
However, the rise of AI also demands that CSMs strike a balance between leveraging technology and maintaining the human touch that fosters trust and long-term customer relationships.
1. Understanding AI Agents and Generative AI
Before diving into applications, it’s important to understand the two key types of AI transforming Customer Success: AI agents and generative AI.
What Are AI Agents?
AI agents are autonomous systems designed to perform specific tasks or workflows with minimal human intervention. They use machine learning, natural language processing (NLP), and other AI technologies to analyze data, make decisions, and execute actions. In the context of Customer Success, AI agents are often used to:
-
Automate repetitive tasks (e.g., sending follow-up emails).
-
Provide real-time insights (e.g., predicting churn risks).
-
Enhance customer interactions (e.g., chatbots for instant support).
What Is Generative AI?
Generative AI, on the other hand, focuses on creating new content (text, images, code, etc.) based on patterns in existing data. Unlike AI agents, which are task-oriented, generative AI is creative and is often used to:
-
Draft emails, reports, or training materials.
-
Generate FAQs, guides, and tutorials.
-
Suggest empathetic responses during customer interactions.
How Do AI Agents and Generative AI Differ?
Aspect | AI Agents | Generative AI |
Purpose | Perform specific tasks or workflows autonomously. | Create new content based on patterns in existing data. |
Functionality | Task-oriented, focused on executing predefined actions or providing insights. | Creative, focused on generating original outputs. |
Examples | Chatbots, predictive analytics tools, automated workflows. | ChatGPT, DALL·E, MidJourney |
Use Case in CS | Automating follow-ups, analyzing customer data, predicting churn. | Drafting emails, creating training materials, generating FAQs. |
Example of using AI Agents Vs Generative AI in HubSpot CRM
Why AI Agents and Generative AI Matter in Customer Success
-
AI Agents handle operational and analytical tasks, enabling CSMs to focus on high-value activities.
-
Generative AI supports creative and communication tasks, enhancing efficiency and personalization.
-
Together, they create a powerful ecosystem for delivering exceptional customer experiences at scale.
2. Key Components of AI in Customer Success
1. AI for Engagement and Personalization
Capabilities Being Used Today:
-
Dynamic Segmentation:
-
Tools like HubSpot CRM and Salesforce Einstein segment customers based on real-time behaviors, such as login frequency, feature usage, and support ticket history.
-
Example: A SaaS company uses Salesforce to identify customers logging in less than twice a month. These customers are automatically enrolled in a re-engagement campaign, resulting in a 25% reduction in churn risk.
-
Impact: Enables hyper-targeted outreach and improves customer retention.
-
-
AI-Driven Chatbots:
-
Platforms like Intercom and Drift use conversational AI to handle routine inquiries, freeing CSMs for high-value interactions.
-
Example: An AI chatbot resolves 80% of common support queries, while escalating complex issues to CSMs. This reduces response times by 40% and improves customer satisfaction.
-
Impact: Enhances efficiency and ensures customers receive timely support.
-
Future Pilots:
-
Dynamic AI Playbooks:
-
Tools like Totango are experimenting with playbooks that adapt in real time based on customer feedback and usage patterns.
-
Potential Benefit: Ensures messaging and strategies remain relevant and effective.
-
2. AI for Predictive Analytics and Risk Management
Capabilities Being Used Today:
-
Churn Prediction Models:
-
Platforms like Gainsight PX and ChurnZero analyze engagement metrics, support tickets, and survey data to flag at-risk accounts.
-
Example: Gainsight detects a drop in feature adoption for a high-value account. The CSM intervenes with a personalized training session, reducing churn risk by 15%.
-
Impact: Proactively addresses issues before they escalate.
-
-
Upsell and Cross-Sell Recommendations:
-
Salesforce Einstein identifies accounts with high potential for premium feature adoption based on usage patterns.
-
Example: Accounts using 80% of their current features are targeted for upgrades, increasing upsell success rates by 20%.
-
Impact: Drives revenue growth while delivering value to customers.
-
Future Pilots:
-
Advanced Predictive Models:
-
Amplitude AI Labs is testing micro-event analysis to detect subtle churn triggers, such as repeated failed logins or ignored emails.
-
Potential Benefit: Enables earlier detection and intervention, further reducing churn.
-
3. AI for Task Automation
Capabilities Being Used Today:
-
Call Transcription and Analysis:
-
Tools like Gong.io and Otter.ai transcribe customer calls and summarize key insights, such as sentiment and action items.
-
Example: Gong flags a negative sentiment during a renewal call and suggests follow-up actions, saving hours of manual analysis.
-
Impact: Enhances decision-making and ensures no critical detail is missed.
-
-
Automated Follow-Ups:
-
Salesforce Workflows generate and send follow-ups based on meeting outcomes or customer actions.
-
Example: After a training session, AI sends tailored resources and a satisfaction survey, improving engagement without manual effort.
-
Impact: Reduces administrative workload while maintaining consistent communication.
-
Future Pilots:
-
Real-Time AI Coaching:
-
Gong.io is developing live coaching tools that suggest empathetic responses and negotiation tactics during calls.
-
Potential Benefit: Helps CSMs navigate high-stakes conversations with confidence and professionalism.
-
Industry Trends and Data
AI Adoption Statistics:
- 72% of organizations are now using AI, up from about 50% in previous years (MCKinsey, 2024) This represents a substantial jump in AI adoption across businesses (MCKinsey,2025)
- 92% of companies are investing in AI, but only 1% have achieved full AI maturity (MCKinsey,2025)
-
By 2028, the chatbot market is expected to reach $15.5 billion, growing at an annual rate of about 23% (Tidio, 2024)
- 79% of companies are using Ai in some capacity in day to day operation (Cledara,2024)
-
Predictive analytics can help SaaS companies forecast monthly recurring revenue (MRR) and optimize pricing strategies (Adelga, 2024)
-
Spotify used predictive analytics to boost customer retention by 15% within six months (Xpandeast,2024)
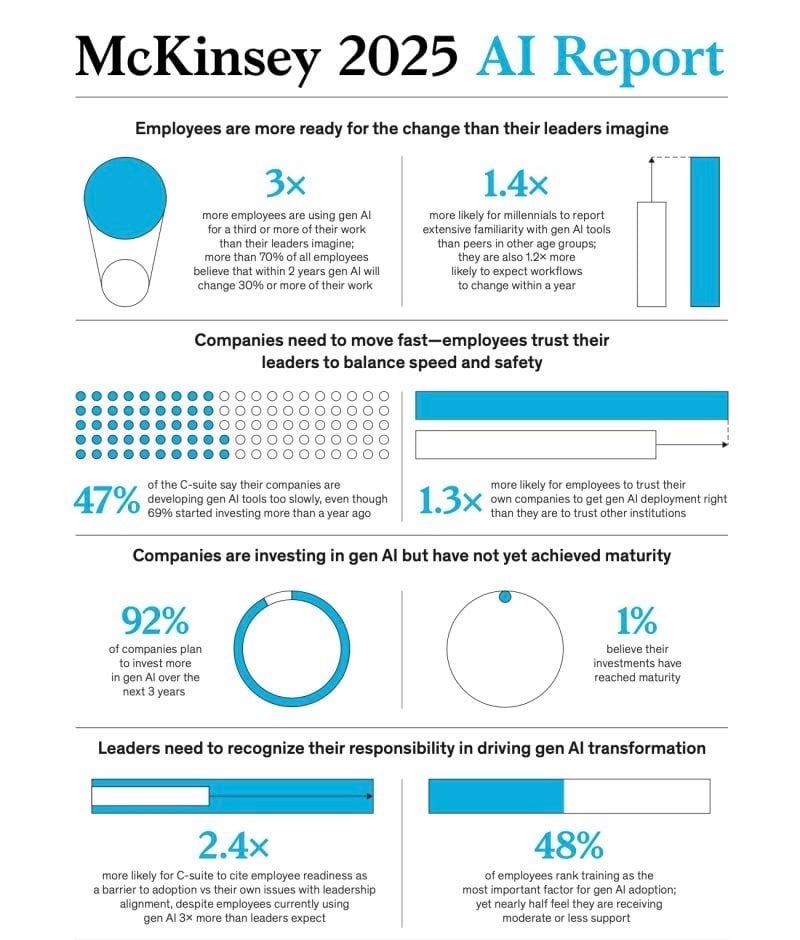
Impact on the CSM Role
-
Larger Book of Business: With AI handling repetitive tasks, CSMs can manage 30-40% more accounts effectively.
-
Improved Churn Prevention: Predictive insights enable proactive retention strategies, improving churn rates by 10-20%.
-
Shift to Strategic Activities: CSMs spend more time on high-value tasks like QBRs, advocacy, and upselling.
- Improved performance tracking: AI-enabled analytics will provide real-time tracking of performance against customer engagement goals, allowing for more precise measurement and improvement of customer success efforts
3. Ethical Considerations in AI Usage
While AI offers immense benefits, it’s important to address ethical concerns to ensure responsible use.
-
Transparency: Clearly communicate to customers when AI is being used (e.g., chatbots, automated emails).
-
Bias Mitigation: Regularly audit AI models to ensure they don’t perpetuate biases in customer segmentation or recommendations.
-
Data Privacy: Ensure compliance with data protection regulations (e.g., GDPR, CCPA) when collecting and analyzing customer data.
Example: A SaaS company uses AI to segment customers but ensures that sensitive data is anonymized and that customers can opt out of data collection.
4. Building an AI-Ready Customer Success Team
Adopting AI tools requires a shift in mindset and skills.
-
Upskilling CSMs: Training CSMs to interpret AI insights and use AI tools effectively.
-
Change Management: Helping teams embrace AI by demonstrating its value and addressing concerns about job displacement.
-
Collaboration with Data Teams: Encouraging cross-functional collaboration between Customer Success and Data Science teams to refine AI models.
Example: A company runs workshops to teach CSMs how to use predictive analytics tools like Gainsight PX and interpret churn risk scores.
5. Integrating AI with Human Touch
While AI can automate many tasks, the human element remains critical in Customer Success.
-
When to Use AI vs. Human Interaction: Use AI for repetitive tasks and data analysis, but rely on humans for complex problem-solving and relationship-building.
-
Augmenting Human Capabilities: How AI can provide CSMs with insights and recommendations to enhance their decision-making.
-
Maintaining Authenticity: Ensuring that AI-generated communications (e.g., emails, chatbots) reflect the company’s tone and values.
Example: A CSM uses AI to draft an email but personalizes it with a handwritten note to add a human touch.
6. Challenges and Future Trends
Challenges:
-
Balancing Automation with Empathy:
-
Over-reliance on AI can lead to impersonal interactions.
-
Solution: Always personalize AI-generated content and ensure human oversight in critical customer interactions.
-
-
Data Quality Issues:
-
Incomplete or inaccurate data limits AI effectiveness.
-
Solution: Regularly audit and clean customer data to ensure accuracy and reliability.
-
-
AI Bias:
-
Models may produce biased outcomes if trained on skewed data.
-
Solution: Continuously evaluate AI outputs for fairness and inclusivity.
-
-
Resistance to Change:
-
Some CSMs may be hesitant to adopt AI tools.
-
Solution: Provide training and demonstrate the value of AI through pilot programs and success stories.
-
Future Trends:
-
Proactive AI Agents:
-
AI will act as co-workers, managing repetitive tasks and providing real-time recommendations.
-
Example: An AI assistant monitors accounts in real time, alerting CSMs when intervention is needed.
-
-
Emotionally Intelligent AI:
-
AI tools will evolve to analyze tone, language, and pacing during interactions, detecting customer emotions more accurately.
-
Example: Real-time coaching tools guide CSMs on how to respond empathetically during difficult conversations.
-
-
AI-Powered Voice Assistants:
-
Tools that can analyze voice calls in real time and provide CSMs with actionable insights.
-
Example: An AI-powered voice assistant analyzes customer calls and provides real-time coaching to CSMs.
-
-
Predictive Playbooks:
-
AI-driven playbooks will adapt to customer behavior and suggest next-best actions.
-
Example: A playbook recommends specific training resources based on a customer’s usage patterns.
-
-
AI for Customer Advocacy:
-
Using AI to identify and nurture potential customer advocates for case studies and referrals.
-
Example: AI analyzes customer feedback to identify advocates who are likely to provide testimonials.
-
7. Future of AI Agents in the Workplace
-
Integrated AI Agents: AI will act as co-workers, managing administrative tasks and alerting CSMs to critical insights.
-
Example: An AI assistant monitors accounts in real time, alerting CSMs when intervention is needed.
-
-
Enhanced Emotional Intelligence: AI tools will evolve to recognize complex customer emotions during interactions, guiding CSMs on how to respond empathetically.
-
Increased Collaboration: AI agents will improve cross-functional collaboration by sharing insights directly with Sales, Product, and Support teams.
Case Study: Reducing Churn and Driving Expansion with AI
Scenario:
Company: A mid-sized SaaS company offering project management software.
Problem: The company is experiencing a 15% churn rate among its mid-tier customers, primarily due to low product adoption and lack of engagement. Additionally, expansion revenue from existing customers is below target.
Framework Applied: The 4-Step AI-Driven Customer Success Framework
-
Identify the Problem: Use AI to pinpoint the root cause of churn and low engagement.
-
Segment and Prioritize: Leverage AI to segment customers and prioritize high-impact actions.
-
Intervene with AI Tools: Deploy AI-driven solutions to address the issues.
-
Measure and Optimize: Use AI to track outcomes and refine strategies.
Step 1: Identify the Problem
-
Data Analysis: The company uses Gainsight PX to analyze customer usage data and identify patterns.
-
Findings:
-
40% of churned customers used less than 30% of the software’s core features.
-
Customers who attended onboarding webinars had a 50% higher retention rate.
-
-
-
Sentiment Analysis: Qualtrics is used to analyze customer feedback from support tickets and surveys.
-
Findings:
-
Customers expressed frustration with the complexity of the software.
-
Many customers were unaware of key features that could solve their pain points.
-
-
Step 2: Segment and Prioritize
-
Dynamic Segmentation: The company uses HubSpot CRM to segment customers based on usage data and engagement levels.
-
Segments Created:
-
At-Risk Customers: Low usage (<30% of core features) and no engagement with training resources.
-
High-Potential Customers: Moderate usage (50-70% of core features) and active engagement with support.
-
-
-
Prioritization: The Customer Success team focuses on:
-
Re-engaging at-risk customers to prevent churn.
-
Upselling high-potential customers to premium features.
-
Step 3: Intervene with AI Tools
-
For At-Risk Customers:
-
AI-Driven Chatbots (Intercom): Deployed to proactively reach out to at-risk customers with personalized messages.
-
Example: “We noticed you haven’t used our task automation feature. Would you like a quick demo?”
-
-
Automated Onboarding Campaigns (HubSpot Workflows): Sent a series of emails with video tutorials and links to upcoming webinars.
-
Personalized Training Sessions: AI tools like Gong.io analyzed past interactions to recommend tailored training topics.
-
-
For High-Potential Customers:
-
Upsell Recommendations (Salesforce Einstein): Identified customers who could benefit from premium features like advanced analytics.
-
AI-Generated Content (ChatGPT): Drafted personalized emails highlighting the value of upgrading.
-
Step 4: Measure and Optimize
-
Outcome Tracking:
-
Churn Reduction: The churn rate among at-risk customers decreased from 15% to 8% within six months.
-
Adoption Improvement: Feature adoption increased by 35% among re-engaged customers.
-
Expansion Revenue: Upsell success rates improved by 20%, contributing an additional $500,000 in annual recurring revenue (ARR).
-
-
Continuous Optimization:
-
The company used Amplitude AI Labs to refine its predictive models, ensuring earlier detection of at-risk customers.
-
Feedback from AI-generated emails was analyzed to improve messaging and personalization.
-
Impact on the CSM Role
-
Efficiency Gains: CSMs saved 10 hours per week by automating routine tasks like follow-ups and data analysis.
-
Strategic Focus: With AI handling repetitive tasks, CSMs spent more time on high-value activities like customer advocacy and strategic QBRs.
-
Improved Customer Relationships: Proactive engagement and personalized support led to higher customer satisfaction scores (NPS increased by 12 points)
Key Takeaways
-
AI Enables Proactive Customer Success: By identifying at-risk customers early, the company was able to intervene before churn occurred.
-
Personalization at Scale: AI tools allowed the team to deliver tailored experiences to hundreds of customers simultaneously.
-
Data-Driven Decision-Making: Insights from AI tools ensured that strategies were based on real customer behavior, not guesswork.
-
Continuous Improvement: The company used AI to measure outcomes and refine its approach, creating a cycle of ongoing optimization.
Practical Exercises
To help learners apply the concepts, here are two hands-on exercises:
-
Exercise 1: Use a free AI tool (e.g., ChatGPT) to draft a personalized email for a hypothetical customer.
-
Exercise 2: Analyze a sample dataset to identify at-risk customers using a predictive analytics framework.